Harnessing the Power of a Video Annotation Tool for Machine Learning
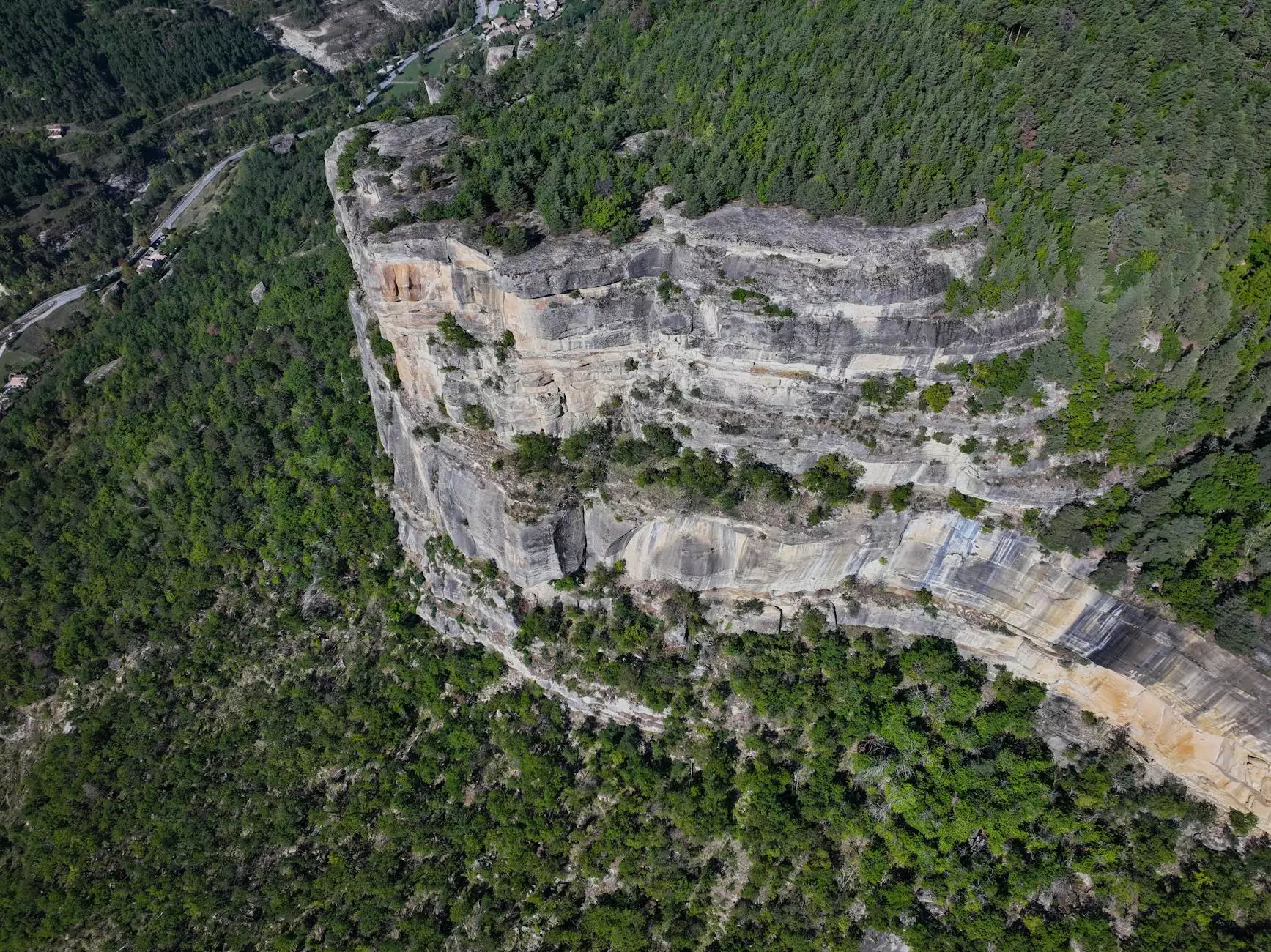
In the rapidly evolving world of artificial intelligence and machine learning, the need for effective data handling and processing is more critical than ever. One of the essential components that power machine learning models is data—specifically, labeled data. A video annotation tool for machine learning has emerged as a pivotal resource for businesses looking to enhance their ML systems while increasing efficiency in data management.
Understanding Video Annotation and Its Importance
Video annotation is the process of labeling and tagging frames in video data to help machine learning algorithms understand and interpret the visual content. This is crucial in a variety of applications, from autonomous vehicles to security systems, and even in healthcare diagnostics.
Why is Video Annotation Important?
- Improved Model Accuracy: Labeled video data allows machine learning models to make precise predictions and decisions, significantly improving their performance.
- Enhanced Object Recognition: With the proper labels, models can better recognize objects and actions within video footage, a necessity for industries like surveillance and retail.
- Action Recognition: In sectors such as sports analysis or healthcare, understanding sequences of actions within videos can provide invaluable insights.
- Automated Processes: By streamlining the annotation process, businesses can automate tasks that traditionally required extensive human input, thus saving time and resources.
The Role of Video Annotation Tools in Business
Businesses across various sectors are increasingly leveraging video annotation tools to meet their specific needs and challenges. Here are some key areas where these tools are making a significant impact:
1. Autonomous Vehicles
Self-driving cars rely heavily on video annotation to train their algorithms. Annotated videos help the AI systems to recognize pedestrians, traffic signs, and other vehicles on the road, enabling safer navigation and decision-making.
2. Healthcare Applications
In healthcare, video annotation tools help analyze medical procedures and patient interactions. For instance, annotating surgical videos allows for the identification of techniques that lead to better patient outcomes, ultimately enhancing surgical training and education.
3. Retail and Customer Insights
Retail businesses use video annotation to analyze customer behavior in stores. Annotated footage can reveal how customers interact with products, allowing businesses to optimize their layouts and improve marketing strategies.
4. Security and Surveillance
Security companies utilize video annotation for surveillance footage to improve incident detection and reporting. Annotated videos can help in identifying suspicious activities and generating actionable intelligence.
Key Features of an Effective Video Annotation Tool for Machine Learning
When selecting a video annotation tool for machine learning, it's essential to consider certain features that can maximize efficiency and effectiveness:
1. User-Friendly Interface
A simple and intuitive interface allows users to annotate videos with minimal training. This efficiency can significantly reduce project turnaround times.
2. Custom Annotation Types
Different projects may require various annotation types, such as bounding boxes, polygons, or semantic segmentation. A versatile tool should accommodate multiple annotation formats to meet diverse project needs.
3. Robust Data Management
Effective data management capabilities are crucial for handling large datasets. A good video annotation tool should enable seamless data organization and retrieval to facilitate easy access to labeled data.
4. Collaboration Features
When multiple team members are involved in the annotation process, collaboration features become invaluable. Tools that enable real-time collaboration can enhance productivity and ensure consistency across annotations.
5. Integration Capabilities
A versatile video annotation tool should easily integrate with existing machine learning frameworks and pipelines. This ability enhances data flow and reduces bottlenecks in the ML development lifecycle.
Implementing Video Annotation in Your Business
Integrating video annotation into your business processes may seem daunting, but with the right approach, it can be streamlined effectively:
1. Define Your Objectives
Begin by identifying the specific use case for video annotation within your organization. Whether it's for training AI models for autonomous vehicles or analyzing customer behavior, clear objectives will guide the project.
2. Choose the Right Tool
Evaluate different video annotation tools for machine learning based on the features discussed above. Opt for a tool that aligns best with your business requirements and budget.
3. Train Your Team
Provide adequate training for your team on how to use the annotation tool efficiently. Ensure they understand its capabilities and best practices for effective video annotation.
4. Monitor and Optimize
As you begin annotating videos, continuously monitor the process. Gather feedback from the team and refine the methods to improve efficiency and accuracy.
Challenges in Video Annotation and How to Overcome Them
While video annotation can significantly enhance machine learning projects, it doesn't come without challenges. Understanding these can help mitigate potential issues:
1. Time-Consuming Process
Annotating videos can be labor-intensive, especially with large datasets. To tackle this, consider using semi-automated annotation tools that can speed up the process by providing initial labeling that humans can refine.
2. Quality Control
Maintaining consistent quality in annotations is crucial. Implement a quality control process where annotations are regularly reviewed and validated to ensure accuracy and reliability.
3. Data Security and Privacy
When dealing with sensitive video data, data security becomes paramount. Choose tools that comply with data protection regulations and ensure that access controls are in place.
Future Trends in Video Annotation for Machine Learning
The future of video annotation tools is promising, with several trends likely to shape the landscape:
1. Increased Automation
Advancements in AI and machine learning algorithms will lead to more sophisticated automated annotation tools. These tools will reduce the workload on human annotators, making the process faster and more economical.
2. Enhanced AI Integration
As AI technology continues to evolve, we can expect deeper integration of intelligent algorithms in video annotation tools, leading to improved accuracy and efficiency in annotations.
3. Cloud-Based Solutions
Cloud technology will further facilitate video annotation, allowing teams to collaborate from anywhere while maintaining access to large datasets without the need for intensive local storage solutions.
Conclusion: Unlocking the Potential of Video Annotation
In conclusion, a video annotation tool for machine learning is not just an asset—it's a necessity for businesses aiming to harness the power of AI. By investing in the right video annotation technology, organizations can improve their productivity while ensuring high-quality data for their machine learning models. This investment will likely lead to better model performance, more insightful analyses, and ultimately, a competitive edge in the market.
As we move into a future that increasingly relies on data and AI, businesses must stay ahead of the curve by adopting effective video annotation methodologies. Embracing these tools will enable organizations to unlock new opportunities for innovation and growth within their respective fields.